A Random Walk With Drift.
Philip A. Etter's Blog
I've finally gotten around to building my mathematics tutorial site. In this site, I will be hosting a number of mathematics tutorials on topics that interest me.
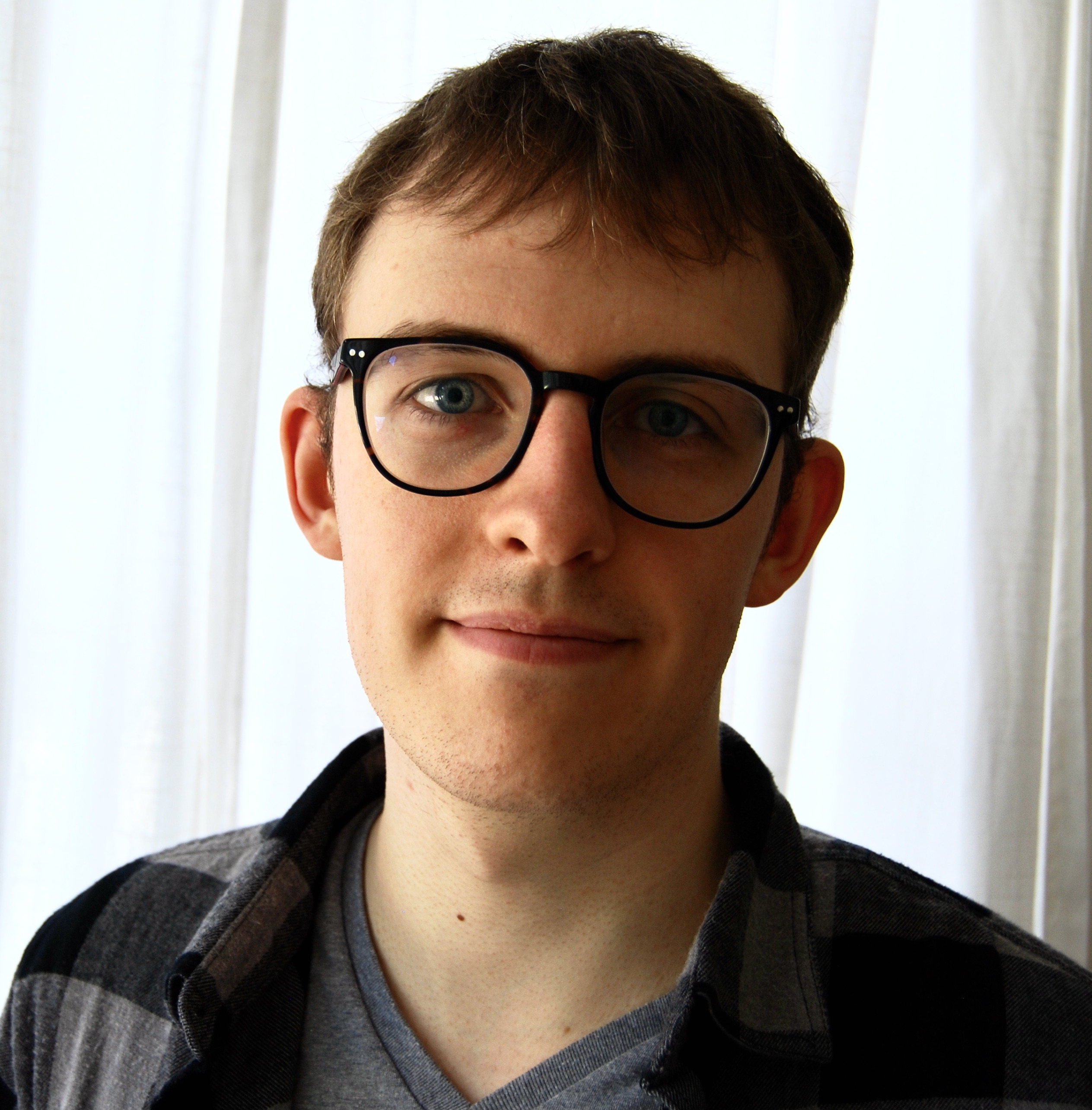
More Stories
Paper: Operator Shifting for General Noisy Linear Operators
This paper is a follow-up paper to my other paper on operator shifting entitled 'Operator Shifting for Noisy Elliptic Systems'. Whereas the previous paper focused on the statistical problem of improving operator inverse estimates in the setting of elliptic (i.e., symmetric positive definite) matrix operators, this paper focuses on the same question when opened to more general matrices. Our approach is to extend the theory and examine if there are any cases where the lack of ellipticity renders the key theoretical results of the previous paper invalid. We identify the three pathological ways that the theory can break down to provide counter-intuitive results: anisotropy, extreme ill-conditioning, and asymmetric small noise. Finally, we show that in the absence of these pathologies one can recover the theory for elliptic operators.
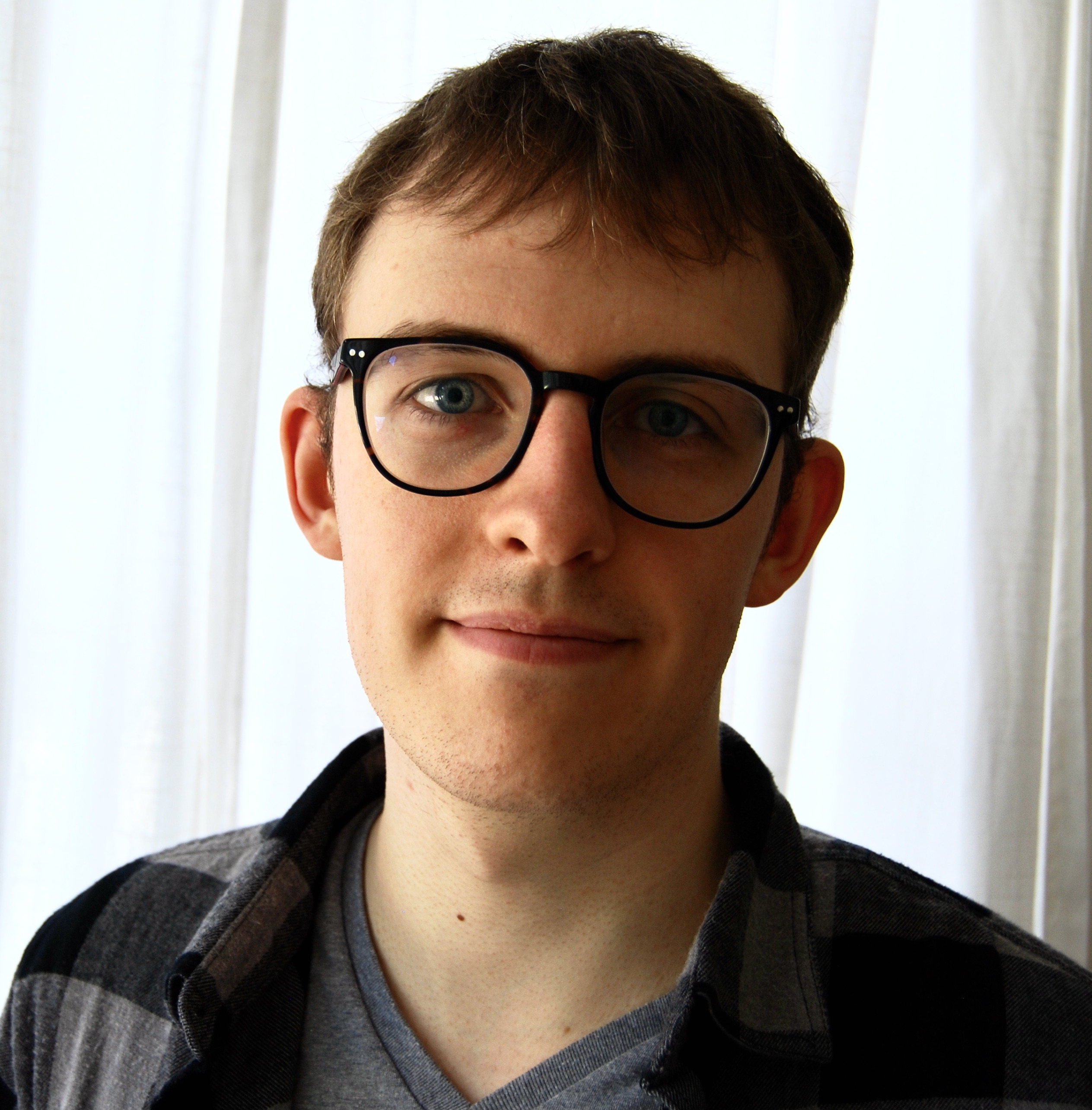
Paper: Operator Shifting for Noisy Elliptic Operators
In the computational sciences, one must often estimate linear model parameters from data subject to noise and uncertainty. In order to improve the accuracy of linear models with noisy parameters, we propose the operator shifting framework, a collection of easy-to-implement algorithms that augment a noisy inverse operator by subtracting an additional shift term. Akin to James-Stein estimation, this reduces both bias and variance in the estimate. We develop bootstrap Monte Carlo algorithms to estimate the required shift magnitude for optimal error reduction. We also provide a set of numerical experiments on four different graph and grid Laplacian systems.
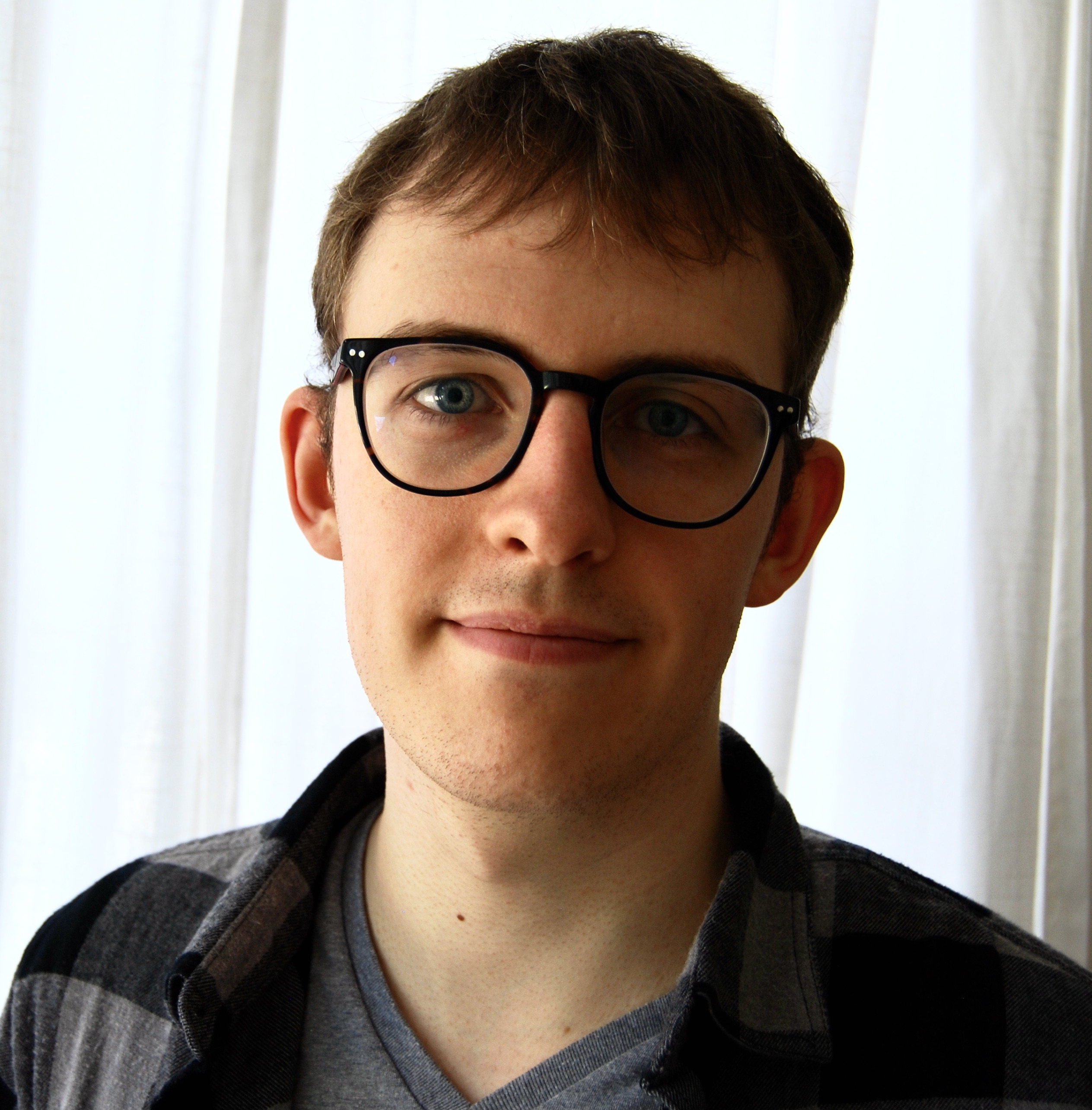
Paper: Accelerating Inference for Sparse XMR Trees
Tree-based models underpin many modern semantic search engines and recommender systems due to their sub-linear inference times. In industrial applications, these models can operate at extreme scales, where every bit of performance is critical. In this paper, we develop a sparse linear algebra technique we call MSCM (masked sparse chunk multiplication). This technique is specifically tailored to sparse extreme multilabel ranking (XMR) trees and consistently dramatic speedups across both the online and batch inference settings. We apply MSCM to an enterprise-scale semantic product search problem with 100 million products and achieve sub-millisecond latency of 0.88 ms per query on a single thread — an 8x reduction in latency over vanilla inference techniques.
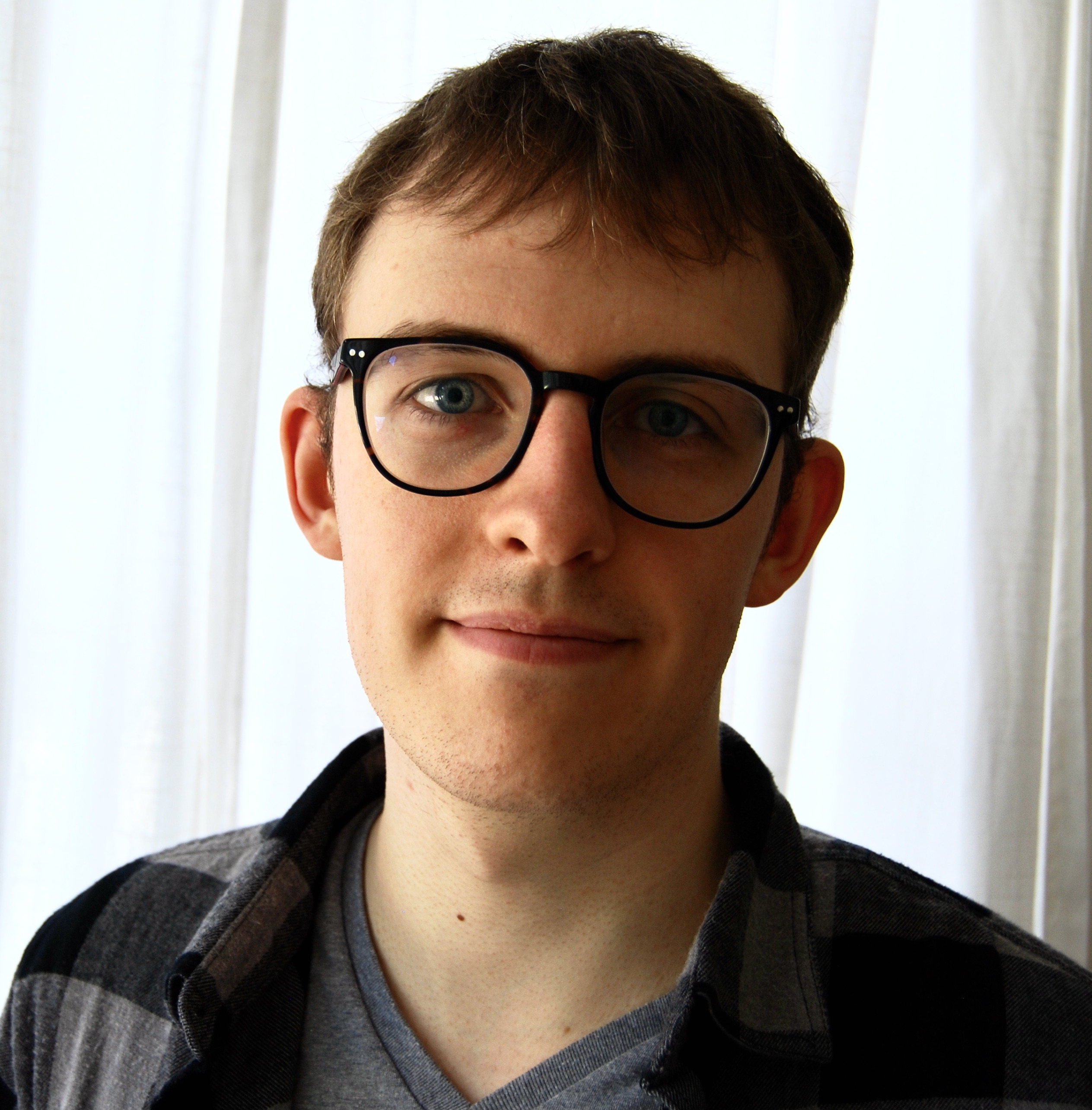
Paper: Adaptive Refinement and Compression for Reduced Models
In this paper, we explore methods to efficiently guarantee accuracy and generalization for linear reduced models even when deployed on out-of-distribution problems. We equip reduced models with both a novel refinement mechanism (vector-space sieving), as well as a fast basis compression scheme; these two innovations together allow us to very quickly adapt a reduced model to new problem distributions. Finally, we demonstrate the effectiveness of our method on several partial differential equation problems.
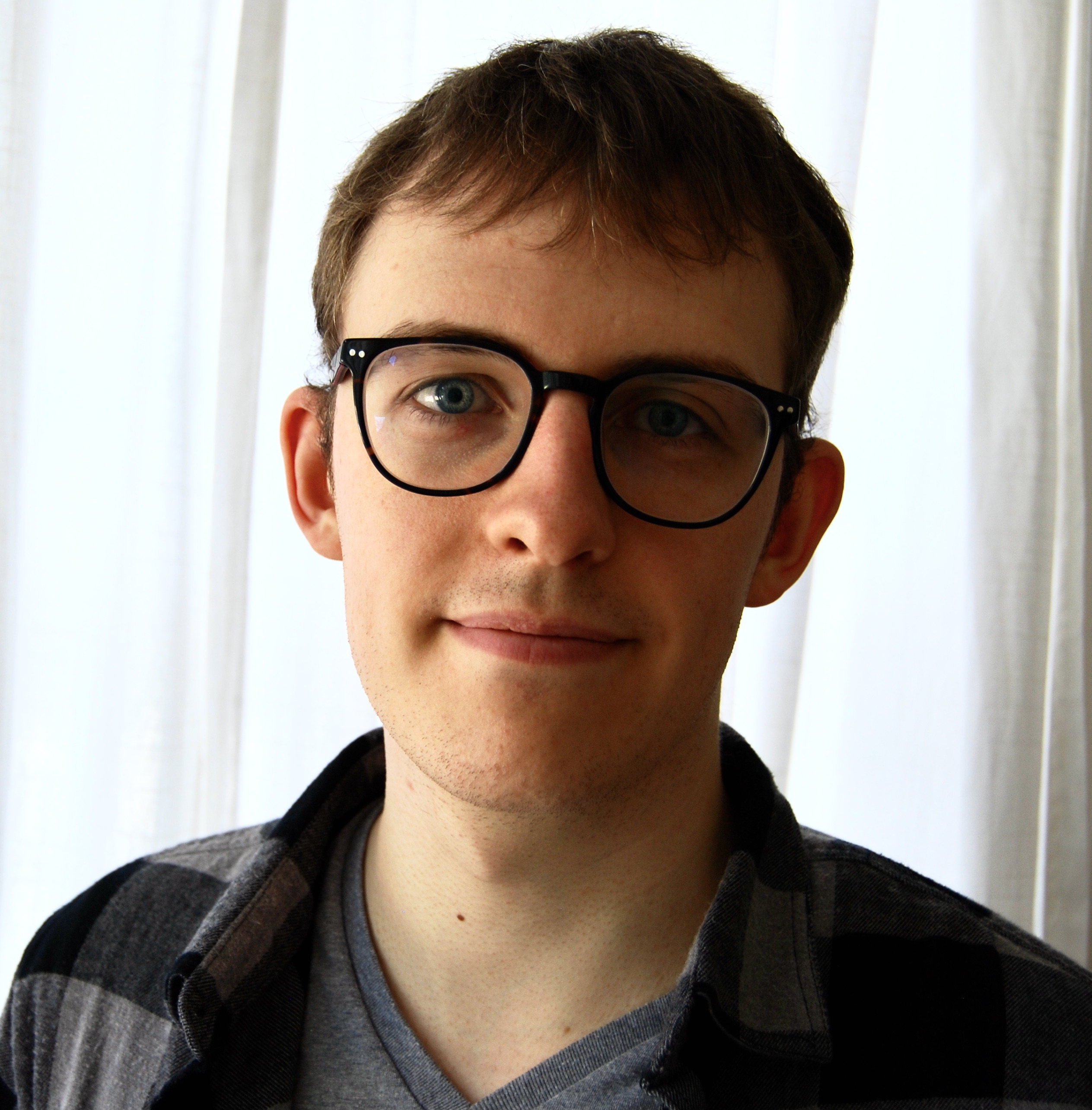
Paper: Coarse Proxy Reduced Basis Methods for Integral Equations
In this paper, we present a novel method for building reduced basis models for integral equations. The method works by using a column-pivoted QR algorithm together with a coarse proxy model to select a skeleton training set and construct a reduced basis from this skeleton set. We combine this with a novel operator interpolation technique specifically designed for integral equations to overcome the typical challenges of model reduction for non-local operators. We demonstrate our results on integral equation formulations of the radiative transport equation and a boundary integral formulation of the Laplace equation.
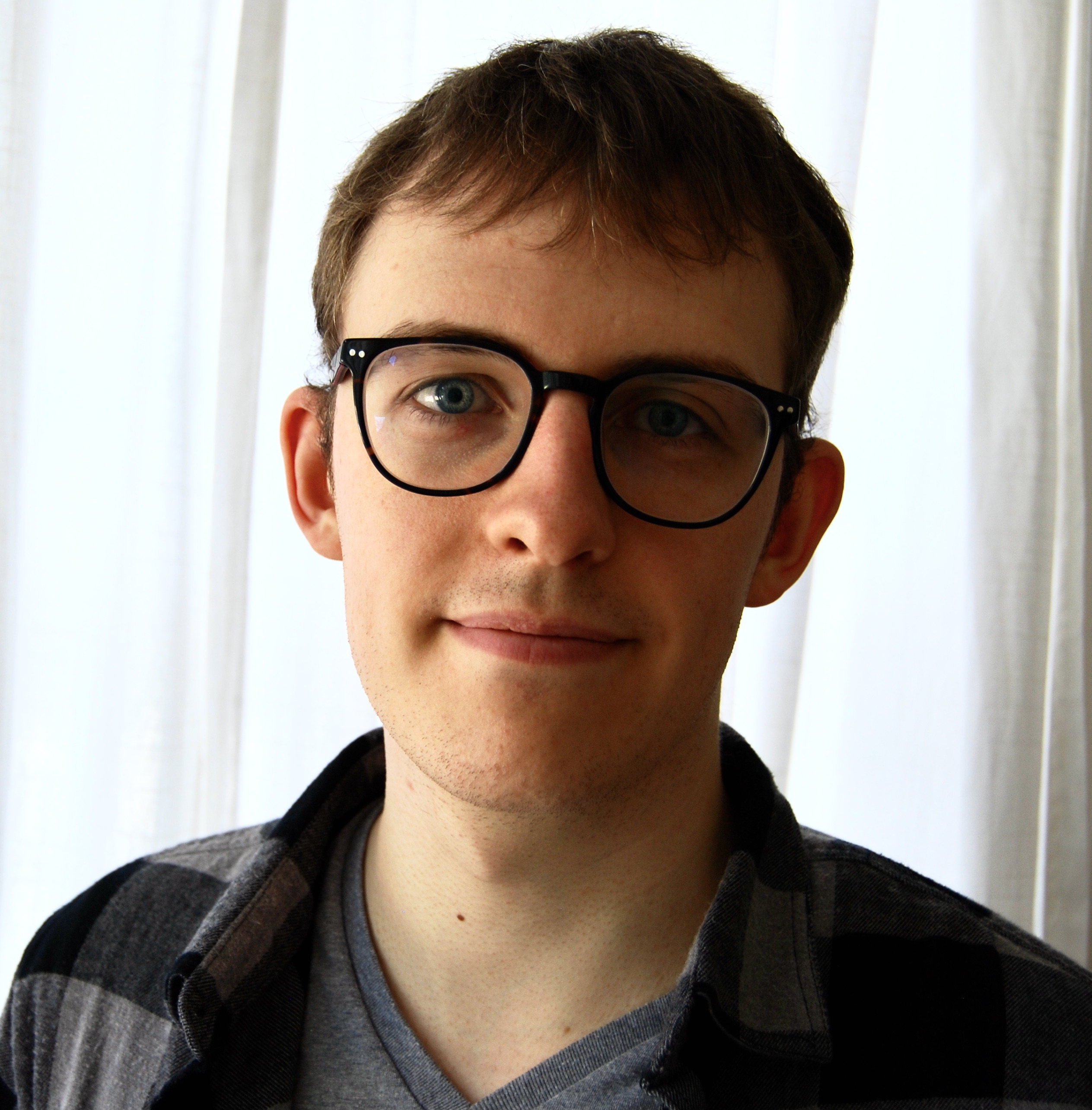